CSCI3320: Fundamentals of Machine Learning
General Expectations:
Student/Faculty's Expectations on Teaching and Learning
Message:
2020-2021 was my last year of teaching CSCI3320, Fundamentals of Machine Learning,
a course which I have taught for 7 or 8 years. It has been fun and as someone said,
usually it is the teacher who learns the most. In any case,
for students who want to take CSCI3320, please refer to the syllabus by the
current course instructor.
Instructor:
Prof. John C.S. Lui , office hours: Thursday, 8:30-10:30am.
Machine learning (ML) is a method of data analysis that automates analytical model building.
Some people say that ML is a branch of artificial intelligence.
Personally, I think that ML is really a branch of statistics.
In any case, this course provides an introduction to machine learning.
It is designed to give undergraduate students
a taste of various machine learning techniques.
Students need to have a good background in
probability, statistics, a bit of optimizaton as well as
programming (e.g., Python) to appreciate various methods.
Furthermore, students need to spend time to read the textbook,
as well as to put in the effort to read various resources on the Internet,
do the homework, attend the lectures and tutorials
to understand and keep pace with this course.
If you skip some classes, please remember that you are solely responsibile for you own actions
on any missed lectures or announcemnets.
Although skipping classes is now a norm in CUHK,
but I like to emphasize that if you skip lectures/tutorial in this course,
you will easily get lost and will not be able to keep pace with the lectures.
So, words of advice, do not skip any classes or tutorials.
Machine learning is an essential knowledge in computer science/engineering,
and a highly sought after skill in the industry.
If you are well-trained in this subject,
surely you can find a good job.
Nevertheless, the subject is
not for the faint-hearted students.
I will discuss the mathematics, theories, algorithms and programming techniques
behind different machine learning
methods, and students need to do various homework and exercises to understand
the subject.
References:
-
Bayesian Reasoning and Machine Learning, by David Barber
-
Pattern Recognition and Machine Learning, by Christopher M. Bishop
-
Machine Learning: A Probabilistic Perspective, by Kevin P. Murphy
-
Learning from Data, by Yaser S. Abu-Mostafa
-
Machine Learning: An Algorithmic Perspective, by Stephen Marsland
-
Machine Learning with R, by Brett Lantz
-
The Elements of Statistical Learning: Data Mining, Inference, and Prediction, by Trevor Hastie, Robert Tibshirani, Jerome Friedman
-
An Introduction to Statistical Learning: with Applications in R,
by Gareth James, Trevor Hastie
-
Mastering Machine Learning With scikit-learn,
by Gavin Hackeling
-
Machine Learning for Hackers, by Drew Conway, John Myles White
-
Probabilistic Graphical Models: Principles and Techniques, by Daphne Koller, Nir Friedman
-
Machine Learning in Action, by Peter Harrington
- Abundant resources available on the web.
Course Grades:
- Written homework (will still be given out): 0%;
- Python/Scikit-learn Programming : 40%;
- Final Examination: 60%
- Policy on letter grades !!!!!
Policies:
Announcemnet:
Final Examination :
Topics to be covered
in the final exam are in general the materials we went through
in the lectures and tutorials, these include:
- Statistics, sampling, curve fitting, correlation theory
- Basic concepts in matrix calculus, linear algebra, Lagrangian Optimization
- Supervised and un-supervised learning
- VC dimension
- Bayesian Decision Theory
- Parametric Methods: Univariate and Multivariate methods
- Dimensionality Reduction via PCA, Feature Embedding, LDA.
- Clustering via K-Mean Algorithm
- EM Algorithm
- Matrix Factorization
- Linear Discriminant: logistic classification and regression
- Decision trees
- Random forests
- Support vector machines
- Neural networks
- ...etc
Lecture Notes:
Lecture and tutorial notes and videos can be downloaded from the Blackboard at CUHK.
Introduction on Machine Learning (online lecture)
Review on Statistics (pre-recorded lecture)
Statistical Sampling
Estimation Theory
Hypothesis Testing
Curve Fitting
Least Sqaured Regression
Regression
Corrrelation Theory
Q-Q Plot
Derivation of Least Squares
Some exercises on "Review of Statistics" (online lecture)
Overview of Supervised Learning (pre-recorded lecture)
What is supervised learning in classification ?
Probably approximately correct (PAC)
Vapnik-Chervonenkis (VC) Dimension
What is supervised learning in regression ?
Examining Your Data or Cleaning your Data: PANDAS Tutorial
(online lecture with Python code in Jupyter notebook)
loading a CSV file
find out various display options
examine the data and schema of the data file
relationship with Python's dictionary
select some features to display
setup a filter and select some data which qualified for the filter
incorporiate the Python's string library to set up a filter
modify feature names as well as data in the dataframe
add/remove data into dataframe
sort data in the dataframe
grouping the data
aggregating the data
exploring the data
casting datatypes and handling missing values
working with dates
working with time series data
reading/writing data to different sources: Excel, JSON, ...etc.
Overview of Bayesian Decision Theory (pre-recorded lecture)
Bayes' Rule: Machine Learning perspective
Loss/Risk Functions, discriminant functions
Introduction to correlation and causality
Introduction to causal and diagnostic inference
Simple Bayesian Networks and Simple Bayes' Classifiers
Association Rules
Regression, Overfitting, Underfitting and Prediction in Python
(online lecture with Python code in Jupyter notebook)
Numpy array
Shape and reshape of Numpy array
Use Numpy array as index on another Numpy array
Elementwise logical comparison in Numpy array
Setting minimum and maximum in all elements in an numpy array via clip()
Cleaning numpy array by filtering NaN entries
Brief introduction to Scipy
Loading datafile via Scipy
Checking NaN and filtering them out via Scipy from the array
Performing a scatting plot in matplotlib
Performing a polynomial best fit on the data
Piece-wise polynomial fit via "one" (or multiple) change point
Fit model after the change point, and use models for "future" prediction
Split the training and testing, and do prediction
Evaluation metrics (pre-recorded lecture)
-
Confusion matrix, accuracy, precision and recall
-
Example code on ML background, confusion matrix, accuracy and recall
(with scikit-learn code in Jupyter notebook)
Data cleansing and data processing in scikit-learn (pre-recorded lecture)
(with scikit-learn code in Jupyter notebook)
-
CSV file as input
-
Data cleansing, re-labelling, one-hot encoding
-
Split and test
-
Decision tree and random forest
Classification in scikit-learn (pre-recorded lecture)
(with scikit-learn code on Jupyter notebook)
-
Decision tree and how it outputs feature importances
-
Display of result using decision tree
-
Use of DummyClassifier and how we loop through different classifier strategy
-
A glimpse of other classifers like: neural network, KNN, SVC, SVM, Linear SVC, Adaboost..etc
-
Concept of training time and score of each classifier
-
Feature importance of Adaboost
-
Multiclass classification
-
Example of digit recognition
-
Confusion matrix and the use of mglearn to display confusion matrix
-
Use of classification_report to display precision, recall, f1-score and suppot for all classes
-
Prediction probabilities for each testing input
Parametric Methods (pre-recorded lecture)
Maximum likelihood estimator
Estimator: bias vs. variance
Unbiased estimator, consistent estimator, asymptotically unbiased estimator
Bayes' estimator
Parametric Classification
Parametric Regression
Bias/Variance Dilemma
Illustration of Model Selection
Introduction to Classification in Python and Scikit-learn
(online lecture with Python and scikit-learn codee in Jupyter notebook)
Visualization of subset of features in our dataset
From visualization, discover classification rules
Use of simple threshold technique to do classification
The need to split up the data into training and validation
From leave-one cross-validation to k-fold cross validation
Using 1NN and KNN as classifiers
The need to normalize all features
Color scatter plot of results in KNN
(with different values of k
Classification via random forest
How to do regression in Python and Scikit-learn
(online lecture with Python and scikit-learn code in Jupyter notebook)
Single feature linear regression (or least square fit)
Multi-dimensional linear regeression
Regression using Ridge, Lasso and ElasticNet Model
Tunning hyperparameter within a learner
Illustrate the problem of not using cross-validation (or use ALL DATA for training).
Illustrate how to use ElasticNet for regresion and how to use the
L1 ratio to tune λ1 and λ2.
Regression in scikit-learn
(pre-recorded lecture with scikit-learn code in Jupyter notebook)
-
load/fetch/make_ datasets in scikit-learn
-
understanding the meta data from a pickel compressed file (PKZ)
-
Regression metrics: explained variance score, mean absolute error, r2 score
-
Doing regression with multiple linear learners
-
Understanding various regularization methods
-
Doing regression with multiple non-linear learners
Real Life Classification: rating answers in Stackoverflow
(online lecture with Python and scikit-learn code in Jupyter notebook)
Fetch and preprocess a 90GB raw XML data (yes, it is painful)
Creating a first nearest-neighbor classifier
Looking into how to improve the classifier's performance
Change from nearest-neighbor to logistic regression
Use precision, recall and AUC to better understand the classifier's performance
Prepare the final version
Dimensionality Reduction (pre-recorded lecture)
Dimensionality Reduction in action
(online lecture with Python and scikit-learn code in Jupyter notebook)
Feature selection vs. feature projection methods
How to use correlation, in particular, Pearson Coefficient, to find out linear relationship among two features
Discuss how to use mutual information to discover linear and non-linear relations between two features.
Discuss how to use recursive wrapper as recursive feature elimination to select features.
Discuss PCA, LDA and Multidimensioal Scaling (MDS).
Clustering (pre-recorded lecture)
Text Pre-processing, NLTK and Finding top k documents via Clustering Technique
(online lecture with Python and scikit-learn code in Jupyter notebook)
Pre-processing documents or text via NLTK, e.g., bag-of-words technique
Compare similarity of a document with a set of documents using raw vectors
Compare similarity of a document with a set of documents using normalized vectors
Applying "stop words" into the vectorizer
Applying "stemming" into the vectorizer
Applying Term Frequency (TF) and Inverse Document Frequency (IDF) into the vectorizer
Applying K-mean algorithm and plotting decision space
Clustering on a realistic dataset
Given a new post, find "similar" posts in a corpus
Multivariate Parametric Methods (pre-recorded lecture)
Multivariate Parameters and Estimation
Multivariate Normal Distributions
Multivariate Parametric Classification in Multivariate Normal Distributions
Multivariate Parametric Classification in Multivariate
Bernoulli/Multinomial Distributions
Multivariate Regression
Linear Discrimination (pre-recorded video)
Generalizing the Linear Model
Geometry of the Linear Discriminant
Linear Discriminant via Pairwise Separation
Logistic Discriminant: Two and Multple Classes
Discriminant by Regression
Discriminant via Ranking
Recommender Systems
(online lecture with Python and scikit-learn code in Jupyter notebook)
Making recomendation in machine learning based on previous user-product ratings (Netflix-like recoomendation)
Visualization of matrix sparsity
Finding similar users or similar products to make recommendation
Using regression technique to make recommendation
Using ensemble learning to make recommendation
Basket analysis for non-numeric data
Apriori algorithm, association rules and their implementation
Nonparametric Methods
(pre-recorded video)
Nonparametric density estimation: Histogram Estimator
Nonparametric density estimation: Kernel Estimator
Nonparametric density estimation: k-Nearest Neighbor Estimator
Nonparametric density estimation: Generalization to Multivarate Data
Condensed Nearest Neighbor
Distance-Based Classification
Nonparametric Regression: Smoothing Models
Decision Trees (pre-recorded video)
Univariate Trees
Prunning on Decision Trees
Rule Extraction from Decision Trees
Learning Rules from Decision Trees
Multivariate Decision Trees
Sentiment Analysis on Tweeter-like data
(online lecture with Python and scikit-learn code in Jupyter notebook)
Learn about Naive Bayes classifier (NBC)
Apply NBC on tweets to do sentiment analysis
Learn various smoothing techniques in "NBC":
(a) Add-one smoothing and, (b) Lidstone smoothing
Learn various performance metrics such as (a) true positive, (b) false positive, (c) false negative and (d) true negative in the confusion matrix.
Extend the performance metircs to: (a) accuracy, (b) error rate, (c) recall, (d) specificity, (e) precision, (f) false positive rate, (g) matthews correlation coefficient, (h) F-score
Basic working principle of Precision-Recall Curve (PRC)
Cleaning the tweets' texts can improve accurac
Use `part-of-speech' (POS) and substitution to refine the classification process
Learn how to use Pipeline mode of data analysis
Learn how to use Grid-search approach to find optimal
values in hyper-parameters
Good video in explaining Area under the Curve (AUC) and Receiver Operator Characteristics (RoC)
Kernel Machines (pre-recorded video)
Quick Review of Logistic Classification/Regression
From Logistic Classification to SVM Classification
Concept of Large Margin
Landmarks to Kernels
Theory of Margin and Support Vectors
Non-separable Case: Soft Margin Hyperplane
Hinge Loss
Kernel Tricks and Kernel Functions
Multiple Kernel Learning and Multiclass Kernel Machines
SVM for Regression
SVM for Ranking
Large Margin Nearest Neighbor
Kernel Dimensionality Reduction
Optional Reading 1:
Constrained Optimization
Optional Reading 2:
Inequality Constraints and Kuhn-Tucker method
Multilayers Perceptrons (Artificial Neural Networks)
(pre-recorded video)
Perceptron
Training a Perceptron
Learning Boolean Functions
Multilayer Perceptrons
Backpropagation Algorithm
Training Procedures
Tuning the Network Size
Bayesian View of Learning
Dimensionality Reduction
Deep Learning
Topic Modeling: Comparing or searching documents by topics instead of words
(online lecture with Python and scikit-learn code in Jupyter notebook)
Learn about the importance of topic modeling and how to search document within a topic.
Learn (at the high level) about latent Dirichlet allocation (LDA)
Learn about gensim package and how to generate topics for corpuses
Learn about visualizing topic distribution and how to use $\alpha$
to vary the distribuiton on associating document to number of topics
Learn about wordcloud package to visualize the words within a topic
Learn about how to find closest topics or documents.
Music Genre Classifiation
(with Python and scikit-learn code in Jupyter notebook (will be uploaded to blackboard))
How to do music genre classification
How to use fast fourier transform (FFT) to convert songs into a vector of numbers, then use these vectors to train our learner
We usee Mel-frequency cepstral coefficients (MFCCs) to convert songs into a vector of numbers, then use these vectors to train our learner
We learn about the phyical meaning of precision/reall curve and ROC curve
We learn how to examine and visualize the confusion matrix
Graphical Models (To be uploaded if time allows)
Conditional Independence
Generative Models
d-Separation
Belief Propagation
Undirected Graphs and Markov Random Fields
Learning Structures from Graphical Model
Influence Diagram
Hidden Markov Models (To be uploaded if time allows)
Discrete Markov Processes
Hidden Markov Models (HMM)
Basic Problems of HMM
Evaluation Problem
Learning the State Sequence
Learning the Model Parameters
The HMM as a Graphical Model
Bayesian Estimation (To be uploaded if time allows)
Bayesian Estimation of Parameters of a Disrete Distribution
Bayesian Estimation of Parameters of a Gaussian Distribution
Bayesian Estimation of Parameters of a Function
Choosing a Prior
Bayesian Model Comparison
Bayesian Estimation of a Mixed Model
Gaussian and Dirichlet Processes, Chinese Restaurants
Latent Dirichlet Allocation
Beta Processes and Indian Buffets
Reinforcement Learning (e.g., Game Theory, Markov Decision Process,..etc.) (To be uploaded if time allows)
Single State Case: K-Armed Bandit
Elements of Reinforcement Learning
Model-Based Learning
Temporal Difference Learning
Partially Observed States
Brief Introduction to Game Theory
Additional References
-
Exploring Python by Timothy A. Budd
-
Think Python: How to Think Like a Computer Scientist, by Allen B. Downey
-
Python Tutorial
-
Python Programming at Youtube
-
Reference note on matrix differentiation
-
Matrix notations and operations
-
Vector notations and operations
-
The Matrix Cookbook by K.B. Petersen and M.S. Pedersen
-
Brief Introduction to Kalman Filters
Tutorial Notes (Availablle on Blackboard)
Tutorial 0: Introduction to Python,
Tutorial 1 (Quick Introduction to scikit-learn with Jupyter notebook);
Tutorial 2 (Review on Linear Algebra And Matrix Calculus, with Jupyter notebook))
Tutorial 3 (Review on Gradient Descent For Linear Regression with Jupyter notebook)
Tutorial 4 (Review on Linear Regression)
Tutorial 5 (Regularization and Cross Validation with Python code)
Tutorial 6 (Parametric Classification and Implementation with sample code)
Tutorial 7 (Principal Component Analysis)
Project Tutorial (Horse Racing Prediction)
Tutorial 8 (Kernel Machines) (To be uploaded)
Tutorial 9 (Ensemble Methods) (To be uploaded)
Homework (Available on Blackboard)
-
Will be posted on Blackboard.
Programming homework
-
Will be posted on Blackboard.
Programming Project :
-
Will be posted on Blackboard.
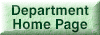